The Language of DNA
Wednesday, 13.09.2023, 15:30 – 16:30
by Anna Poetsch
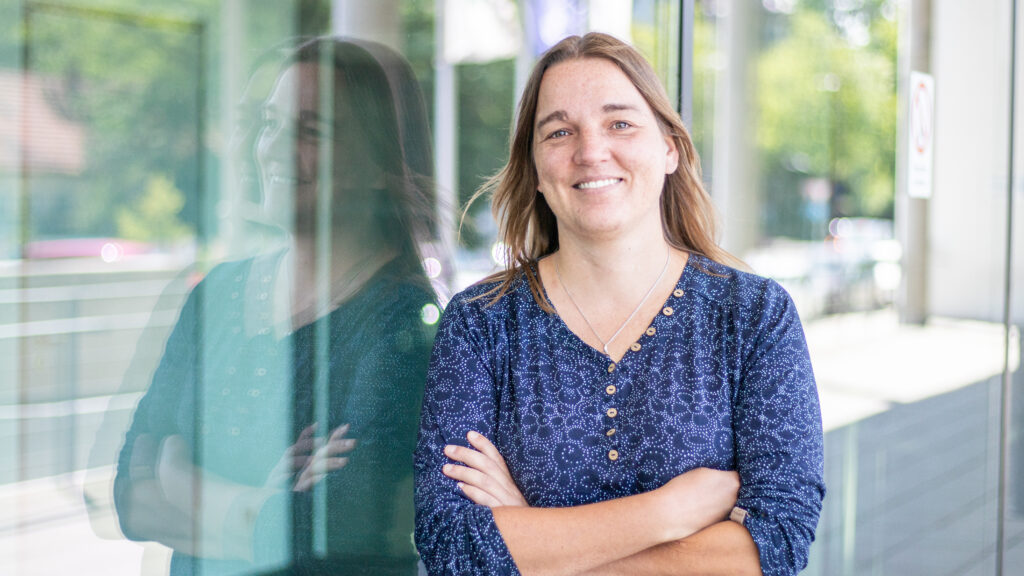
Anna Poetsch moved to Dresden in July 2020. She spent her postdoctoral time at the Francis Crick Institute with a placement to the Okinawa Institute of Science and Technology (OIST).
She did her PhD at the German Cancer Research Institute (DKFZ) and undergraduate training at University Konstanz, the Japanese National Cancer Center Research Institute, and ALTANA Pharma AG.
Anna’s background is in classical biochemistry/ molecular biology, DNA damage response, and mutations in cancer. Her interest in the associated processes has not changed, but the methodology has become increasingly computational, deeper and deeper into deep learning.
Abstract
The human genome is the blueprint to build an entire human being, yet how the 3 billion letters of the human genome achieve this, is still rather poorly understood. 1-2% of the genome encodes for proteins, the molecular machines and building blocks of our body, but the genome encodes much more. For example, it encodes how the protein coding parts are regulated, and also the genome’s own stability, i.e. which positions are prone to mutation and breakage. Since such processes are very important for development and treatment of cancer, we would like to understand how these codes work together and interact.
We have adapted methodology from natural language processing (NLP) to build a DNA language model, GROVER (Genome Rules Via Extracted Representations). Analogous to models for natural language, the foundation model learns the language rules of the genome. With fine-tuning tasks and other techniques, we can now extract what the model has learned and thus formulate relationships between the “words” in our genome. We can also use the foundation model to formulate fine-tuning tasks analogous to natural language. For example, a TextToImage task would be analogous to GenotypeToPhenotype, which links genetic variation to specific traits like eye colour or clinical outcomes. What Translation is for natural language allows us to translate genomic data over different species, or personalize human genomic data from the reference genome to an individuum or an individual cancer. Generative AI allows us to impute data, increase depth and resolution of genome data analysis.
However, as with natural language, we are also facing biases based on the data we are training with. Most current NLP models are trained on the internet with all its racism, sexism and hegemonic viewpoints. How can we use analogous strategies in genomics without doing the same mistakes? Genome research benefits already preferentially people who are close to the human reference genome. Are we integrating the same biases? Can we actually use DNA language models to actively work against them?
We have adapted methodology from natural language processing (NLP) to build a DNA language model, GROVER (Genome Rules Via Extracted Representations). Analogous to models for natural language, the foundation model learns the language rules of the genome. With fine-tuning tasks and other techniques, we can now extract what the model has learned and thus formulate relationships between the “words” in our genome. We can also use the foundation model to formulate fine-tuning tasks analogous to natural language. For example, a TextToImage task would be analogous to GenotypeToPhenotype, which links genetic variation to specific traits like eye colour or clinical outcomes. What Translation is for natural language allows us to translate genomic data over different species, or personalize human genomic data from the reference genome to an individuum or an individual cancer. Generative AI allows us to impute data, increase depth and resolution of genome data analysis.
However, as with natural language, we are also facing biases based on the data we are training with. Most current NLP models are trained on the internet with all its racism, sexism and hegemonic viewpoints. How can we use analogous strategies in genomics without doing the same mistakes? Genome research benefits already preferentially people who are close to the human reference genome. Are we integrating the same biases? Can we actually use DNA language models to actively work against them?